Problem: Use image classification to determine locations of land cover classes within Black Water National Wildlife Refuge, Maryland (Fig. 1).
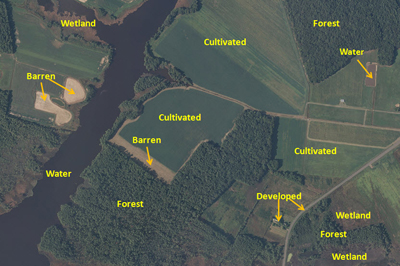
Figure 1. Image of Black Water National Wildlife Refuge for classification with example land cover categories (provided by NCSU GIS 520).
Analysis Procedures: Image classification is used to simplify complex imagery (e.g. aerial orthophotos, satellite images, etc.) into discrete categories. The user identifies areas for each category, the software compares the pixel values of the user-identified areas to the remainder of the image, and results in a classified final image (Fig. 2). Raster images of the area of interest should be obtained in a fine enough resolution that land cover types can be visually identified. If the classification will include vegetation, one should consider using raster data that include the near-infrared band, as this will increase the contrast between vegetation and other land cover categories. Several small polygons are selected from homogeneous areas of a land cover class. These polygons are merged within the training sample manager into a single class. The process is repeated for each land cover category. Scatterplots for each land cover class and image band are then examined. Areas of overlap indicate poor separation of classification and/or similar land cover characteristics. Supervised classification is then run and the resulting classified image is compared to the original raster data. The classified image is inspected for areas of misclassification, training polygons are adjusted or added, the scatterplots can be re-examined, and the supervised classification re-run. This process is repeated until the results are satisfactory. A procedure log for this exercise is available here.
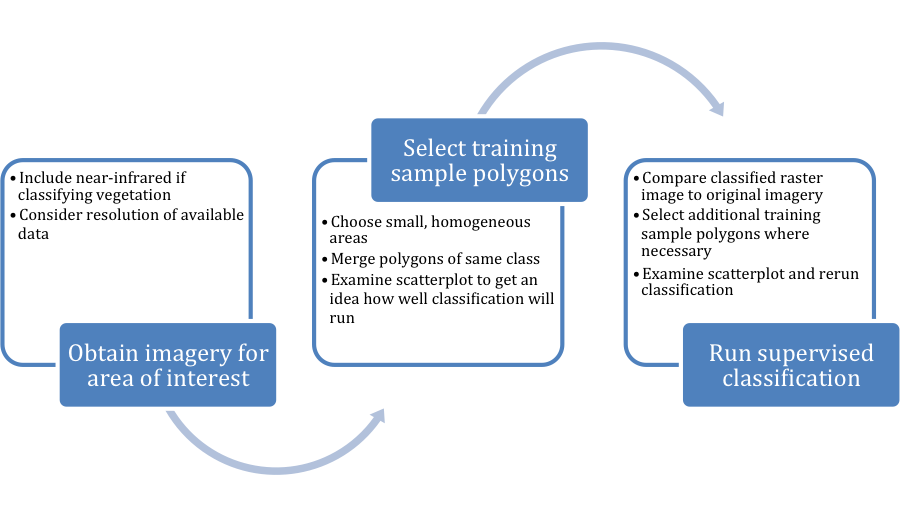
FIgure 2. General workflow diagram for image classification procedure. Click on figure for enlarged image.
Results:
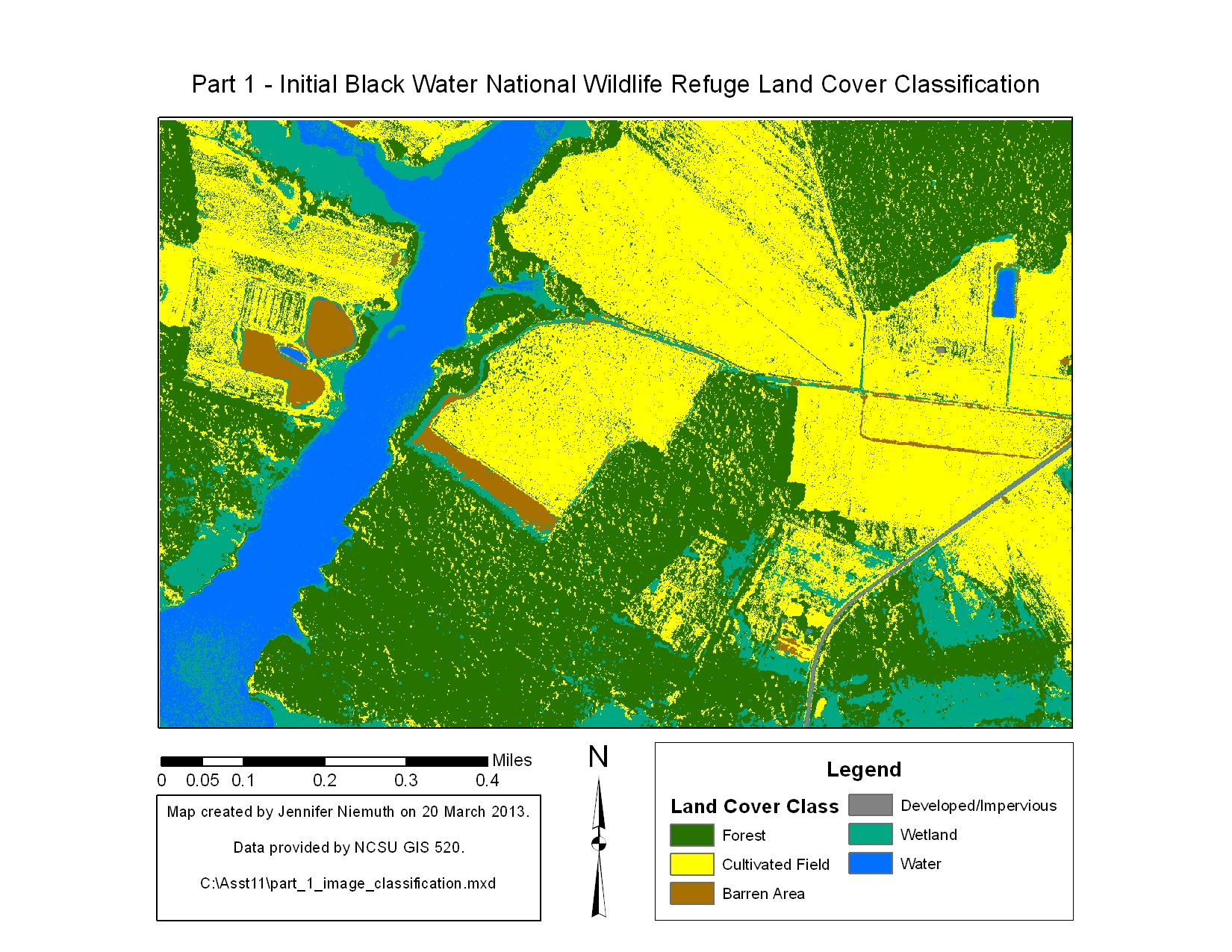
Figure 3. Results of image classification after initial sample training polygon selection (click here to see a map of the polygons selected and click here to view the classification scatterplots). Note that some areas of open water are incorrectly classified as wetland, cultivated fields contain many forest pixels, and the shadows in some of the cultivated fields are classified as wetland. Click on map for enlarged image.
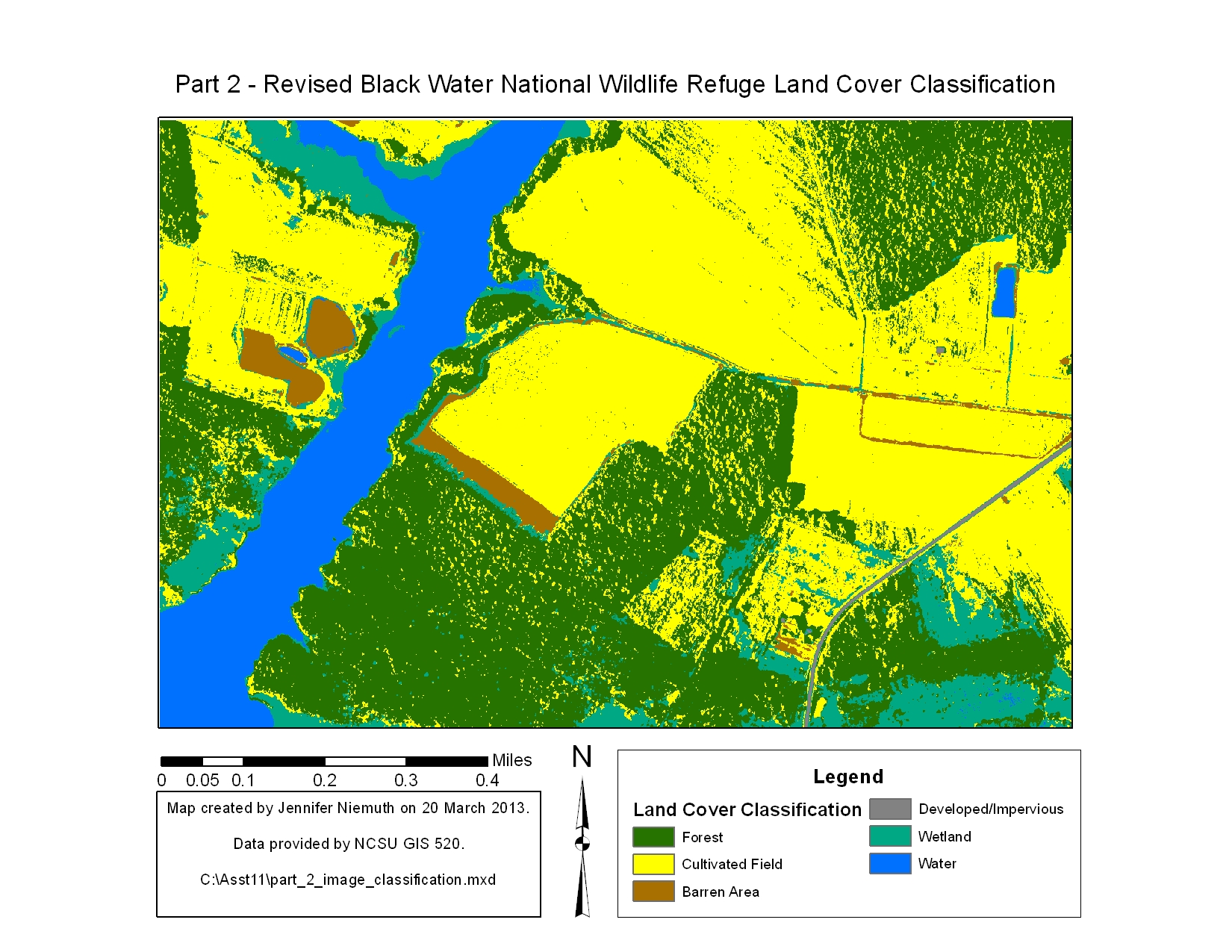
Figure 4. Revised image classification (click here to see the revised training polygons, click here to see a map of the revised scatterplots). Note that the areas of incorrectly classified water have been corrected and the cultivated fields are more homogeneous. However, the new polygons resulted in greater overlap of the cultivated field and forest categories. Attempts to correctly classify the shadowed regions resulted in severe errors in classification across the rest of the map. Click on map for enlarged image.
Application and Reflection: The skills and concepts learned in this exercise can be used in a variety of applications. Image classification can be used for wildlife ecology as a measure of habitat fragmentation, forestry as a monitor of succession, agriculture to check crop health, and in many other fields. Some imagery can even be used for image classification and surveillance of invasive species of aquatic plants or coral reef habitats (Fig. 5).
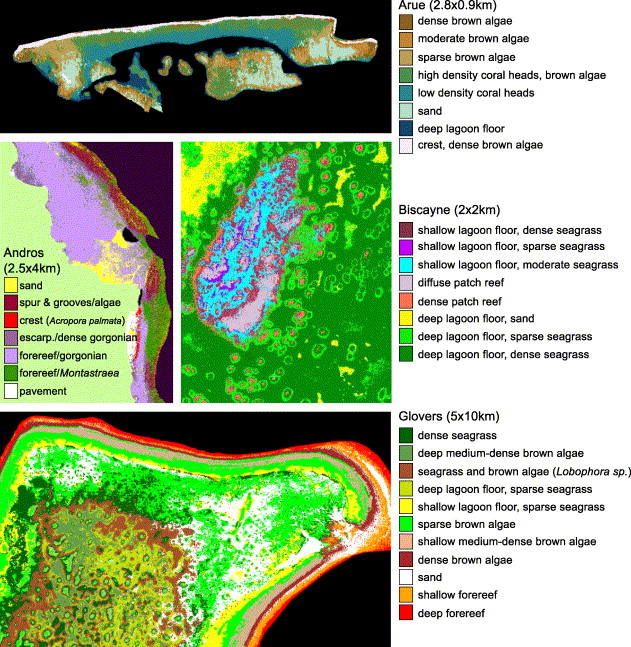
Figure 5. Andréfouët et al. (2003) showed that a combination of IKONOS and Landsat imagery could be used to classify coral habitats. These are examples of classification maps for Arue, Andros, Biscayne, and Glovers reef sites.